Part of the use to which I’d like to put this blog is to disseminate information about research methods and tools. But before I start writing posts with involved details it’s probably prudent to present some sort of overview of the whole thing. Of course, there is no single method that is used by all computer scientists, although each method usually tries to approximate the scientific method as closely as possible. Hence, what I have to talk about is not the method utilized by all researchers, but it is a common one in the sub-field of free/open source and software evolution.
It was, I think, Daniel German who first suggested the role of a software evolutionist — a kind of palaeontologist, or private investigator, of software. Like a detective or an archaeologist, the software evolutionist arrives at the scene. Before her is a program listing, thousands of lines long. She doesn’t know how it got to be in the state she finds it, but clues may be available for her to piece its development together.
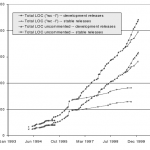
Besides the code, there’s the support documentation (maybe that will tell her how the program is meant to function). Also open on the computer is a forum where all the developers communicate (perhaps this will shed some light on what the developers were assigned). And on the server is a version control system, a treasure trove of clues that shows exactly which developers did what, and when they did it.
Unlike the detective we’re not trying to find a murderer of course, but we are trying to piece together how the program developed over time, i.e. how it evolved. An early example of this was done by Michael Godfrey and Qiang Tu: with nothing but a load of historical releases of the Linux kernel between 1994 and 1999, they showed that the kernel grew at a super-linear rate (it grows by a larger amount as time goes by) and identified which parts of the kernel were responsible for this surprising growth. (Spoiler: the portion of the kernel that contains device drivers was the biggest driver of this growth.)
So how do software evolutionists do it? As I said I can’t speak for them all, but I’ll try to articulate an abstract version of the steps that I and others go through, and assume it approximates the experience of the rest.
Roughly speaking the typical steps involve:
- Selection: Both of the project to study and the measures you wish to apply;
- Retrieval: Getting hold of the software (not always easy!) and storing it appropriately;
- Extraction: Parsing the raw data, extracting the pieces you are interested in, and constructing them into useful information;
- Analysis: Applying the measures and performing your relevant test(s).
In later posts of this category I’ll discuss the tools and techniques of each stage, and (hopefully) build up a picture of the method. For now, I’ll show trivially how an analysis of the Linux kernel size might fit in with this approach (taking cues from Godfrey and Tu’s study where possible).
- Selection: The Linux kernel is selected as a large exemplary open source project. Because the size is the attribute of interest, the number of lines of code is taken as a measure of size. To be scientific we should form some testable hypotheses predicting what we expect to find.
- Retrieval: Each kernel version release is available on the Linux Kernel Archives as a tar file. Godfrey and Tu downloaded 96 of the releases.
- Extraction: Now the lines of code (LOC) are counted in each release. Godfrey and Tu applied the Unix command “wc -l” to all *.c and *.h files and used an awk script to ignore non-executable lines.
- Analysis: By this point, there should be 96 numbers stored, each the size of a release in LOC. To get a visual, we can feed them into a plotting program and produce a nice graph like the one above. We could even go further and apply all sorts of fancy mathematics or models. Suffice it to say, by the end of this stage we should have some results that allow us to confirm or refute our earlier hypothesis.
Once all this is done, we can then put forward out conclusions. Like a scientific study, the experimental data we have obtained is the evidence that backs them up.
You are talking my language. You might find some of the discussion about workflows in this (and earlier OSSmole) papers interesting:
http://floss.syr.edu/publications/HowisonTavernaDemoIFIP.pdf
Also, I noted the change of your blog url; will the blog still be picked up by FLOSS planet blog? Hope so, since that’s the way I read it.
Hi James, thanks for the sustained interest! Gregorio has amended FLOSSPlanet so I should be back on there now.
Enjoyed the paper, some great points about how FLOSS research should be improved. I hope to become more proactive in this once my current work is completed (thesis write up) and I can get back to some research again.